Πυροδοτούμενα νευρωνικά δίκτυα και εφαρμογές τους
Spiking neural networks and applications
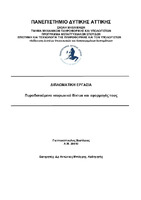
Μεταπτυχιακή διπλωματική εργασία
Author
Γιαννακόπουλος, Βασίλειος
Date
2021-12-21Advisor
Bogris, AdonisKeywords
Spiking neural networks ; Robotics ; Memristor ; Photonics ; Plasticity ; Visual place recognition ; Machine learning ; Liquid state machine ; Visual computing ; SpintronicsAbstract
Πρόσφατα επιστημονικά δεδομένα από την επιστήμη της νευρολογίας μας έχουν δείξει ότι οι
νευρώνες κωδικοποιούν πληροφορία μέσω του χρονισμού των ηλεκτρικών πυροδοτήσεων που
προκαλούνται και όχι από την συχνότητα που πυροδοτούνται. Έτσι μας δίνεται η αφορμή για την
διερεύνηση αυτής της προσέγγισης όσο αφορά τα νευρωνικά δίκτυα στο κομμάτι της
πληροφορικής. Αρχικά γίνεται μια εισαγωγή όσο αναφορά την μηχανική μάθηση, τους τρόπους
εκπαίδευσης και τα προβλήματα που επιλύουν. Στην συνέχεια γίνεται μια προσέγγιση στα
νευρωμορφική υπολογιστική, ως παρακλάδι της μηχανικής μάθησης με αναφορές στις
υλοποιήσεις από πλευράς hardware(memristors, photonics) , με τελικό προορισμό τα spiking
neural networks(Πυροδοτούμενα νευρωνικά δίκτυα) στα οποία θα αναφερθούν μοντέλα αυτών,
μέθοδοι training(εκπαίδευσης) καθώς και μερικά χαρακτηριστικά που πρέπει να τα διέπουν
(plasticity).Τέλος γίνεται αναφορά σε εφαρμογές αυτών στην καθημερινότητα(αναγνώριση
εικόνας, ρομποτική) και σε μελλοντικά βήματα όσο αναφορά την περαιτέρω ανάπτυξή τους.
Abstract
Recent scientific data from the science of neurology have shown us that neurons encode
information through the timing of the electrical triggers caused rather than by the frequency they
are triggered. This gives us the opportunity to explore this approach as far as neural networks in the
field of information technology are concerned. Initially, an introduction is made as far as reference
is made to machine learning, the ways of training and the problems they solve. Then there is an
approach to neuromorphic computing, as an offshoot of machine learning with references to
implementations in terms of hardware (memristors, photonics), with final destination to spiking
neural networks (Spiking neural networks) that will be mentioned, methods of training (supervised,
reward modulated, reinforced) as well as some characteristics that should govern them (plasticity).
To sum up we propose applications for SNN at visual Computing and robotics and some future
steps as far as their further development.