Prediction of the retention time of natural product metabolites using transfer learning strategies
Πρόβλεψη του χρόνου κατακράτησης μεταβολιτών φυσικών προϊόντων με χρήση στρατηγικών μεταφοράς μάθησης
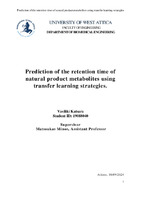
Keywords
Metabolomics ; Retention time ; Molecular fingerprints ; Molecular descriptors ; Transfer learning ; Machine learningAbstract
Retention time (RT) prediction in chromatography can play an important role for numerous analytical applications, including drug discovery and environmental monitoring. This study aims to enhance RT prediction accuracy by employing deep learning techniques, particularly focusing on transfer learning to adapt models trained on synthetic compounds acquired by High Pressure Liquid chromatography–Mass Spectrometry (HPLC-MS) to predict RTs for natural products in different chromatographic methods. We utilized the extensive METLIN Small Molecule Retention Time (SMRT) dataset, comprising over 80,000 synthetic compounds, to train a deep neural network (DNN). This model was then fine-tuned on smaller datasets of natural products, from the RepoRT database using a two-stage transfer learning approach. Initially, the DNN’s upper layers were frozen to retain knowledge about high level features while training on the new data. Subsequently, all layers were unfrozen for further training with a reduced learning rate, ensuring both general and unique patterns were captured. Hyperparameter optimization was conducted using Optuna, leveraging a 5-fold nested cross-validation to ensure robust performance. The evaluation metrics that we computed were the Mean Absolute Error (MAE), the Median Absolute Error (MedAE) and Mean Absolute Percentage error (MAPE). Transfer learning was then compared with new trained DNNs directly trained on the RepoRT database and showed that the strategy was successful according to the MAE and MadAE metric, although not according to the MAPE. We decided to remove the outliers and noticed that with the cleared data transfer learning performed better considering all the metrics. In the future, it will be necessary to refine this strategy to improve its performance, either by testing it on the same datasets or by incorporating additional data.